In today’s data-driven world, businesses have access to a wealth of information. However, the true value doesn’t just come from collecting data, but from uncovering meaningful insights. This is where data mining for business intelligence comes into play, as a powerful tool for discovering hidden patterns, trends, and connections within extensive datasets. But, what exactly is data mining and how does it impact business intelligence?
What is Data Mining?
Data mining is the process of analyzing large datasets to uncover valuable insights and knowledge. As of 2021, North America holds the largest market share in the data mining industry. It involves a combination of statistical analysis, machine learning algorithms, and database techniques to sift through massive amounts of data and identify patterns or relationships that may not be immediately apparent. Essentially, it’s like mining for diamonds in a rough – extracting valuable gems of information from a sea of raw data.
How does Data Mining for Business Intelligence work?
When we talk about analyzing data, data mining for business intelligence plays a pivotal role. The process typically begins with defining the objectives of the analysis – what specific questions do we want to answer or problems do we want to solve? Then, we gather data from different sources like databases, APIs, and other repositories.
Once the data is collected, it undergoes a series of preprocessing steps to clean and prepare it for analysis. This involves removing irrelevant or duplicate entries, handling missing values, and transforming the data into a suitable format for analysis. With the data in hand, business intelligence uncovers patterns, trends, or relationships within the dataset.
Methods of Data Mining for Business Intelligence
Data mining includes various methods and techniques, each tailored for different types of analysis. Now, let’s explore some of these methods:
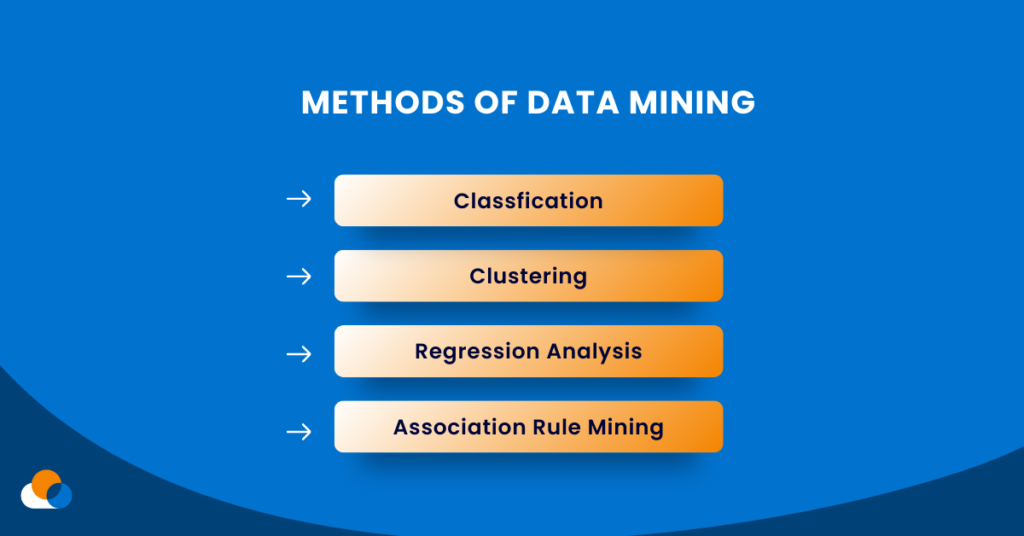
Classification:
Classification involves assigning predefined labels to new data based on existing patterns. This method is often used for tasks like spam email detection, sentiment analysis, and credit scoring.
Clustering:
Clustering involves grouping similar data points based on shared characteristics. This method is useful for tasks like customer segmentation, anomaly detection, and market segmentation.
Regression Analysis:
Regression analysis is used to predict numerical values based on variables in the dataset. This method is commonly used for sales forecasting, demand prediction, and price estimation.
Association Rule Mining:
Association rule mining involves identifying relationships between variables in large datasets. This method is often used for tasks such as market basket analysis, recommendation systems, and cross-selling strategies.
These methods, along with others like anomaly detection and text mining, enable businesses to extract valuable insights from their data and drive actionable intelligence.
Ways to Apply Data Mining for Business Intelligence to Businesses
Data mining applications for business intelligence are diverse and can be used in different ways. Let’s explore some common ways businesses leverage data mining:
1. Market Basket Analysis:
Market basket analysis involves analyzing customer purchase patterns to optimize product recommendations and cross-selling opportunities. For example, a grocery store might use market basket analysis to identify items frequently purchased together, such as chips and salsa, and then promote them as a bundle.
2. Customer Segmentation:
Customer segmentation entails grouping customers based on shared characteristics or behaviors to tailor marketing strategies and improve customer satisfaction. An e-commerce platform might segment customers based on their purchase history, demographics, or browsing behavior to deliver personalized recommendations and promotions.
3. Predictive Analytics:
Predictive analytics utilizes historical data to forecast future trends and outcomes, enabling businesses to make proactive decisions and mitigate risks. For instance, a retail chain might use predictive analytics to forecast demand for certain products based on seasonal trends and economic indicators, optimizing inventory management and pricing strategies accordingly.
4. Fraud Detection:
Fraud detection involves identifying suspicious activities or anomalies in financial transactions to prevent fraudulent behavior and mitigate risks. Financial institutions use data mining techniques to analyze transaction patterns and detect unusual behavior, such as unauthorized account access or fraudulent transactions, in real-time.
Examples of Businesses Leveraging Data Mining for Business Intelligence
There are several industries that utilize data mining’s capabilities, to achieve an advantage and boost growth. For example:

1. Healthcare and Medical Institutions
Healthcare organizations are increasingly turning to data mining for business intelligence to improve patient outcomes, optimize treatment plans, and reduce healthcare costs. By analyzing electronic medical records, clinical data, and patient demographics, healthcare providers can identify patterns and trends that help in diagnosing diseases earlier, predicting patient outcomes, and personalizing treatment plans. For example, data mining techniques can be used to analyze patient data to identify factors that contribute to readmissions, allowing hospitals to implement targeted interventions and reduce healthcare costs.
2. Manufacturing and Supply Chain Management
Data mining for business intelligence is an important tool in manufacturing and supply chain management as it helps businesses identify inefficiencies, optimize processes, and improve product quality. Manufacturers can use data mining techniques to analyze production data, identify bottlenecks, and optimize production schedules to meet demand more efficiently. Additionally, data mining can be used to analyze supply chain data to identify trends and patterns that help businesses optimize inventory levels, reduce costs, and improve overall supply chain efficiency.
3. Telecommunication Companies
Telecommunication companies leverage data mining for business intelligence to analyze customer usage patterns, identify trends, and predict customer churn. By analyzing call detail records, customer demographics, and usage patterns, telecommunication companies can identify customers at risk of churn and implement targeted retention strategies. For example, data mining techniques analyze customer usage patterns to identify customers who are likely to switch to a competitor and offer them targeted promotions or discounts to retain their business.
4. Transportation and Logistics
Transportation and logistics companies use data mining for business intelligence to optimize route planning, improve fleet management, and reduce transportation costs. By analyzing historical transportation data, weather patterns, and traffic conditions, transportation companies can optimize routes, minimize fuel consumption, and reduce delivery times. For example, data mining techniques can analyze historical delivery data to identify inefficiencies in delivery routes and optimize routes to reduce transportation costs and improve delivery times.
5. Hospitality and Tourism Industry
The hospitality and tourism industry utilizes data mining to analyze customer preferences, improve customer satisfaction, and optimize pricing strategies. Hotels and resorts can use data mining techniques to analyze booking patterns, customer reviews, and demographic data to identify trends and preferences among different customer segments. For instance, data mining techniques can analyze customer reviews to identify common complaints or issues and implement targeted improvements to enhance customer satisfaction and loyalty.
6. Financial Institutions
Financial institutions rely on data mining for business intelligence to detect fraudulent activities, assess credit risks, and enhance customer experience. By analyzing transaction data, customer demographics, and market trends, financial institutions can identify patterns and anomalies that help detect fraudulent transactions and prevent financial losses. For example, data mining techniques analyze transaction patterns to identify unusual behavior, such as large withdrawals or transfers, that may indicate fraudulent activity. Additionally, data mining can assess credit risks by analyzing customer credit histories, payment behavior, and financial profiles, allowing financial institutions to make more informed lending decisions and manage risk effectively.
7. Retailers
Retailers use data mining for business intelligence to analyze consumer behavior, optimize inventory management, and enhance marketing strategies. According to Zipdo, retailers can increase their operating margins by up to 60% using data mining for business intelligence. By analyzing sales data, customer demographics, and purchasing patterns, retailers can identify trends and preferences that inform product assortment decisions and promotional activities. Data mining techniques can analyze sales trends to determine the most profitable products, optimize pricing strategies, and personalize marketing campaigns to target specific customer segments.
8. E-commerce Platforms
Data mining for business intelligence helps E-commerce platforms personalize user experiences, improve product recommendations, and optimize conversion rates. By analyzing user behavior, browsing history, and purchase patterns, e-commerce platforms can tailor product recommendations and marketing messages to individual customers, enhancing user engagement and driving sales. Data mining techniques can analyze customer browsing behavior to identify products of interest and deliver targeted recommendations, increasing the likelihood of conversion and repeat purchases.
9. Energy and Utilities Sector
Energy and utility companies leverage data mining to optimize energy production, improve grid reliability, and reduce costs. By analyzing data from smart meters, sensors, and other sources, energy companies can identify trends and patterns that help optimize energy production and distribution. This sector utilizes data mining for business intelligence by analyzing energy consumption patterns to identify opportunities for energy conservation and efficiency improvements, reducing costs and environmental impact.
How does WebDataGuru make Data Mining for Business Intelligence easier?
Now that we know the various ways data mining for business intelligence can benefit, it’s only natural to ask where to begin and if it’s as time-consuming as it sounds. Businesses can enjoy numerous advantages by using a data mining service such as WebDataGuru, having several years of data mining experience for a diverse set of customers. One of the main benefits is the ability to tap into a vast amount of data without the need to gather and analyze it on your own. This not only saves time and resources but also guarantees the accuracy and dependability of the acquired data.
Book a demo with us today and access essential data for market research and strategy optimization.
Recent Comments